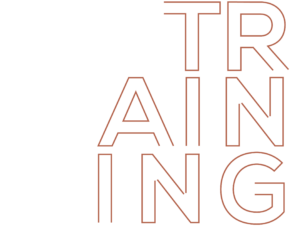
Digital Sport Sciences
The digital revolution in sports and exercise is already underway and creates a demand for skilled professionals able to bridge the gaps between the different scientific fields covered by DIGISPORT :
sport science, computer science, data science, electronics, human and social sciences.
OBJECTIVES OF THE TRAINING PROGRAM
The Digital Sport Sciences master degree is based on research and innovation to train professionals capable of :
- Apprehend the challenges of the digital transformation of sport regarding performance, health, physical activity
- Develop a cohesive vision of the principal scientific and technical issues that arise from the integration of new technologies in sport and exercice, from their use as well as structuring changes and innovations they bring
- Master the concepts, methods, tools, et technological know-how supporting the current development of this field
The Digital Sport Sciences master degree delivered by DIGISPORT focuses on modularity, allowing students to tailor their training program in accordance with their professional project. The master is divided into majors and minors during the first three semesters. The 4th and last semester is dedicated to the end-of-studies intership.
The major (90 ECTS), includes core courses mandatory for all students as well as specialisation courses to elect according to the student’s targeted work profile.
The minor (30 ECTS) enables the acquisition of complementary skills in another field and allow students to build a unique profile tailored to their aspirations and competences. It also includes a project course, allowing students to develop their research, interdisciplinarity and collaboration skills.
THE DIGITAL SPORT SCIENCES MASTER DEGREE
delivered by DIGISPORT
is built on a competency-based approach.
These competencies can be grouped into 4 different interdiscplinary profiles.
PROFILE
Metrology of human movement and innovative sensors
PROFILE
Analysis, modeling & simulation
of human mouvement
PROFILE
Data science
applied to sport
PROFILE
Digital solutions for
interaction in sport
Structure of the master degree
INTERNSHIPS
Students have to complete two periods of interships in a company or laboratory during their training program.
FIRST INTERNSHIP
the first intership period must be
completed during the 2nd semester (Year 1).
SECOND INTERNSHIP
the second intership period must be
completed during the 4th semester (Year 2).
Examples of interships performed by DIGISPORT students :
- Monitoring and modeling of performance in swimming
- Biomechanical and physiological adaptations to novel sensori-motor congruences during virtual cycling
- Assessment of a VR-based training tool for the optimization of perceptual-motor processes of boxers
- Sport video contents quality assessment
- Embedded active vision for body-localization in learning-based swimming gesture analysis